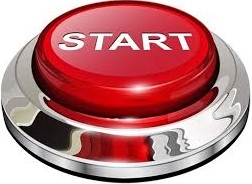
The next data science step is the dreaded data preparation process that typically takes up to 80% of the time dedicated to a data project. If you're working on a fun project outside of work, these open data sets are also an incredible resource! Step 3: Explore and Clean Your Data A lot of countries have open data platforms (like in the U.S.).
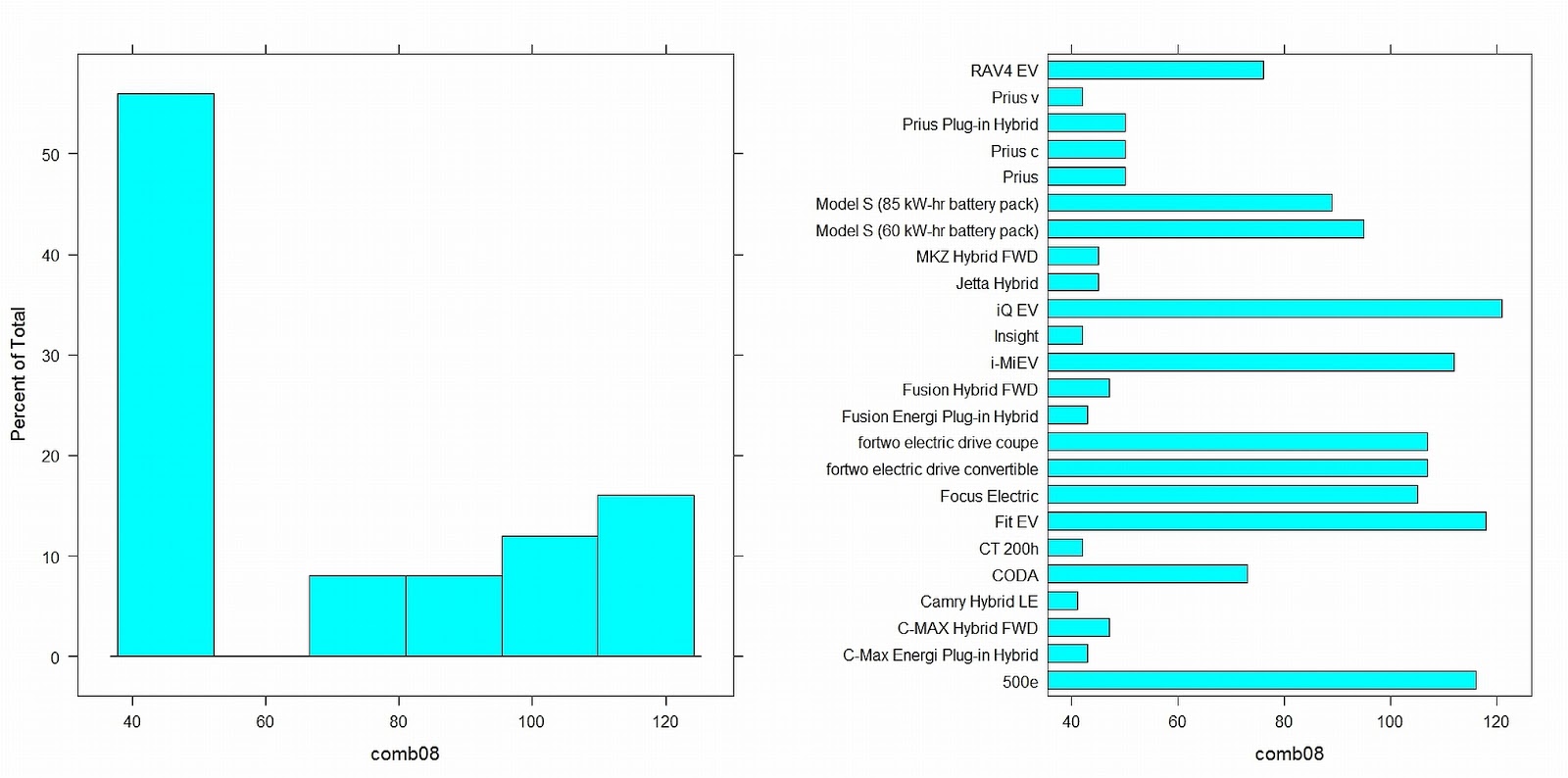
For example, census data will help you add the average revenue for the district where your user lives or OpenStreetMap can show you how many coffee shops are on a given street.
Basic data analysis full#
Look for open data: The Internet is full of datasets to enrich what you have with additional information. If you’re not an expert coder, plugins in Dataiku give you lots of possibilities to bring in external data! You have to work on getting these all set up so you can use those email open and click stats, the information your sales team put in Pipedrive or Salesforce, the support ticket somebody submitted, etc. Use APIs: Think of the APIs to all the tools your company’s been using and the data these guys have been collecting. Here are a few ways to get yourself some usable data:Ĭonnect to a database: Ask your data and IT teams for the data that’s available or open up your private database and start digging through it to understand what information your company has been collecting. Mixing and merging data from as many data sources as possible is what makes a data project great, so look as far as possible. Once you’ve gotten your goal figured out, it’s time to start looking for your data, the second phase of a data analytics project.
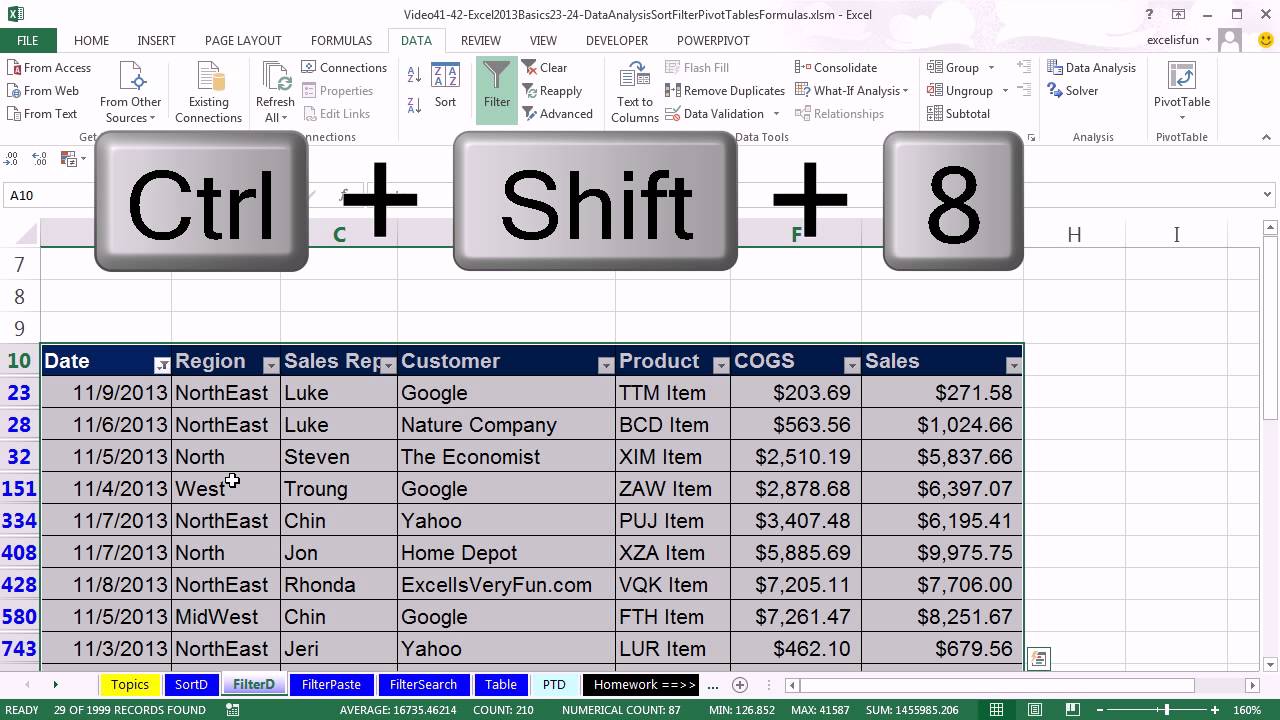
In order to have motivation, direction, and purpose, you have to identify a clear objective of what you want to do with data: a concrete question to answer, a product to build, etc. Simply downloading a cool open dataset is not enough. If you’re working on a personal project or playing around with a dataset or an API, this step may seem irrelevant. I know, planning and processes seem boring, but, in the end, they are an essential first step to kickstart your data initiative! Then, sit down to define a timeline and concrete key performance indicators. Before you even think about the data, go out and talk to the people in your organization whose processes or whose business you aim to improve with data. To motivate the different actors necessary to getting your project from design to production, your project must be the answer to a clear organizational need. Understanding the business or activity that your data project is part of is key to ensuring its success and the first phase of any sound data analytics project. The following is our take on a data project definition via the fundamental steps of a data analytics project plan in this exciting age of AI, machine learning, and big data! These seven data science steps will help ensure that you realize business value from each unique project and mitigate the risk of error.
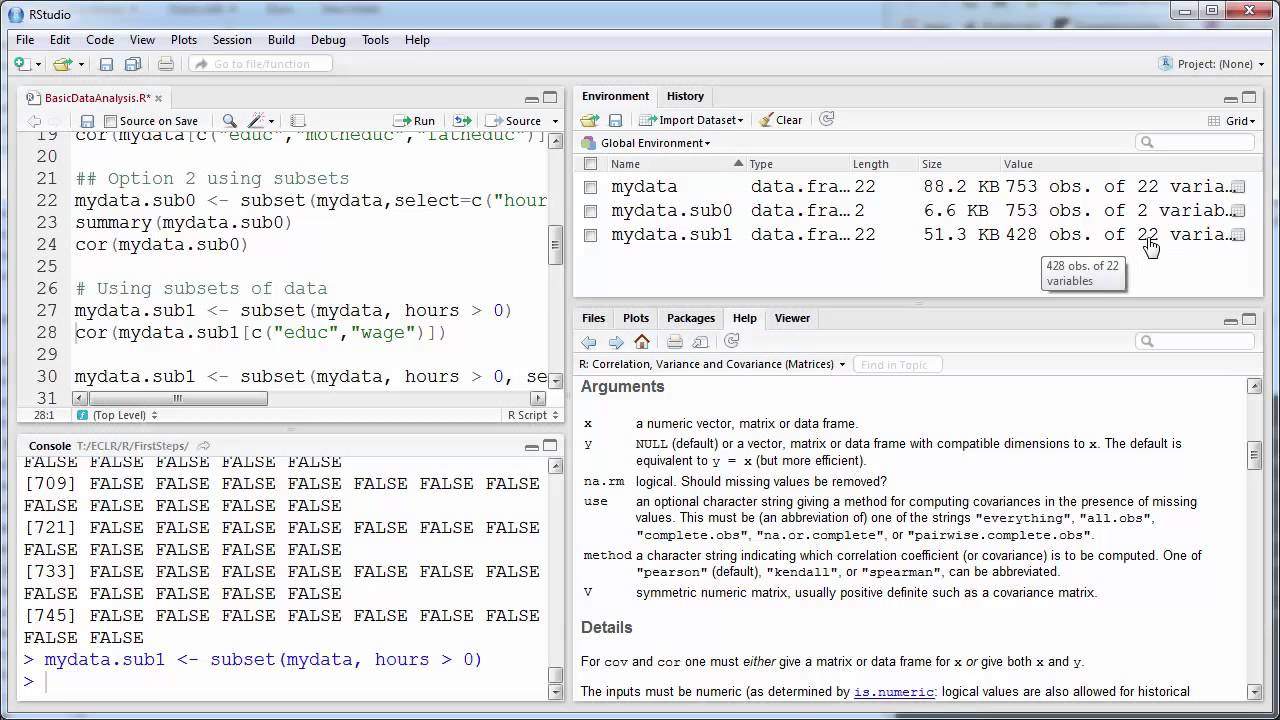
Becoming data-powered is first and foremost about learning the basic steps and phases of a data analytics project and following them from raw data preparation to building a machine learning model, and ultimately, to operationalization.

Yes, starting with a tool that is designed to empower people of all backgrounds and levels of expertise such as Dataiku helps, but first you need to understand the data science process itself. Luckily for you, building your first data analytics project plan is actually not as hard as it seems. What data science steps do you take first? Just looking at all the technologies you have to understand and tools you’re supposed to master can be dizzying. It's hard to know where to start once you’ve decided that, yes, you want to dive into the fascinating world of data and AI.
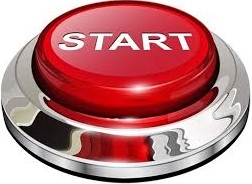